Stop chasing the p-value
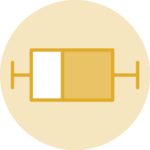
You just spent hours analyzing data and got a p-value of 0.051. Does that make your findings meaningless? Would 0.049 really change everything?
This post explains why chasing statistical significance often misses the bigger picture. Practical significance matters more.
๐ค Comparing Two Groups
โ Data and Question
You have values for two groups. Calculating the mean and variance for each group is straightforward.
But are the differences statistically significant? Can we conclude that the groups are meaningfully different, or could the observed differences be due to random chance?
โ T-Test, p-Value, and Effect Size
The t-test is a statistical method designed precisely for this purpose. The result is a p-value, which indicates the probability of observing the data if the null hypothesis (no difference between groups) is true.
๐ฎ Visualizing the p-value
The sliders below let you experiment with sample size, effect size(average difference), and standard deviation. The values of both groups are displayed on a boxplot using jittering to illustrate their distribution.
Adjust the sliders to build an intuition about how the p-value changes!
For example, consider this scenario. With a standard deviation of 4
and an effect size of 2
, the difference won't be statistically significant with a sample size of 25
. However, it will become significant if the sample size is increased to 50
!
Explore how the p-value behaves.
Conclusion
In summary, increasing the sample size will always make a difference statistically significant eventually. But does that mean it truly matters? Sometimes yes, sometimes noโit depends on the context.
Statistical tools like the p-values are valuable for analyzing data, but they're just one part of the bigger picture.
Focusing solely on whether a result is "statistically significant" can lead to misleading interpretations and overlook the practical importance of findings.
By considering effect sizes, confidence intervals, and the context of your data, you can draw conclusions that are not only statistically sound but also meaningful and impactful.
Stop chasing the p-value. Start seeking the story behind the numbers!
Contact
๐ Hey, I'm Yan and I'm currently working on this project!
Feedback is welcome โค๏ธ. You can fill an issue on Github, drop me a message on Twitter, or even send me an email pasting yan.holtz.data
with gmail.com
. You can also subscribe to the newsletter to know when I publish more content!